Data vs. the digital display
- Patrick Van Hull
- Apr 27, 2023
- 3 min read
Updated: Apr 29, 2023
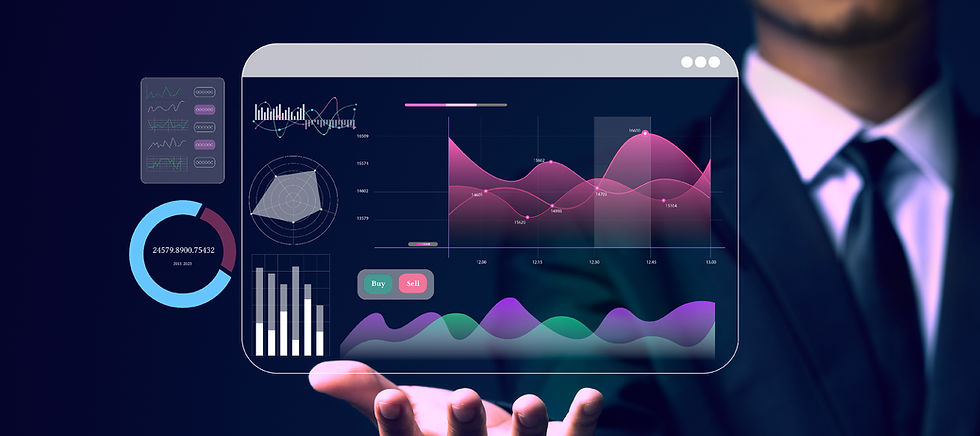
The red digital numbers on top of the machine identified the operating speed. The production supervisor was adamant about that until we pressed him for data. He used hand-written log sheets of runtime and pallets of finished products as proxy metrics in crudely calculating the run rate.
While we felt frustrated that we couldn’t validate the data, its absence supported our proposal to explore using innovative tools to provide better insight into overall equipment effectiveness (OEE). At the core of our message was that by analyzing actual data, the company could identify bottlenecks in the supply chain and work to eliminate them.
Our small team of two data scientists and I set out to pilot using computer vision in that nearly 60-year-old manufacturing facility. We started with handheld Go-Pro cameras aimed at various spots on the machines to create sample videos and dashboards. The clips said more than we thought possible.
In one video, we saw finished goods get stuck at the machine’s output, destroying a significant volume of products. Another video showed finished goods accumulating beyond the allotted storage space, causing production downtime.
We knew we had the beginnings of a good business case, but the challenges were extensive. We needed to mount the camera so it wouldn’t be disturbed or blocked. We needed a reliable power source. We needed reliable network connectivity and mass storage for video streaming.
The computer vision pilot ultimately proved two things: there were problems worth solving, and a different method was a more practical solution. The company chose to use sensors and other connected devices in an Internet of Things (IoT) approach to measuring OEE, tracking the location and condition of their inventory, and reducing waste.
Initially, we set out to show that data could help identify bottlenecks and improve efficiency. Had we lost sight of the objective, we could have pushed too hard and gone too far down the wrong path. We ultimately got there in a different way than anticipated but saved everyone the rework costs, financial and otherwise, that add up far too quickly.
It’s easy to call the project a failure, but that neglects the importance of the lessons learned.
Even if we think we see the correct data, whether on a display or dashboard or hand-written log, it’s worth looking periodically to validate the information. Sure, that means taking added time to verify data sources and connectivity, but that small cost must be considered part of the risk mitigation plan.
The newest, flashiest technology isn’t always the right choice. Companies embracing a transformation mindset can build a roadmap to create world-class next-generation capabilities. However, the starting point often needs to be simple. Smaller-scale improvements can build resiliency by upgrading beyond ad-hoc band-aids.
Use the early stages of projects and initiatives to gather data while iterating. Beginning with the end in mind is a known success factor, but that doesn’t mean being overly attached to initial plans and hypotheses. Qualitative data can be more telling than initial quantitative data at the beginning, so record observations and note additional questions to ask. Constantly evaluate if the processes and promises align with the business's most important storylines.
Let me know when you’re ready to discuss your strategy for upgrading your capabilities. Book a meeting with me to explore the right path and work together to shape your impact.
About the Author
Patrick Van Hull
Chief Storyteller at Supply Chain Storyteller